GPU servers for PyTorch offer specialized computing environments designed to accelerate the training and inference phases of deep learning models. These servers are equipped with high-performance GPUs, making them perfect for researchers, developers, and data scientists working with PyTorch, one of the most popular deep learning frameworks.
Whether for academic research, enterprise applications, or personal projects, selecting the right GPU server can drastically reduce development time and improve model performance. Here, we highlight five of the best GPU servers tailored for PyTorch applications, each offering unique features and capabilities to cater to a variety of needs and budgets.
Table of Contents
The Top 5 Best GPU Servers for PyTorch
1. GPU-Mart
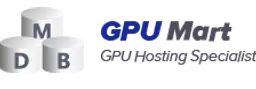
- Supports over 19 GPU card models
- Optimized for high-performance computing tasks
- 24/7 technical support
- Comprehensive list of supported GPU applications
- Starting at $21.00/month
- Starting at $21.00/month
Pros
- Flexible server customization options
- Accepted as ideal for deep learning apps like PyTorch
- Cost-effective hosting solutions
- Dedicated and reliable GPU server rental
Cons
- Proper cost management required
- Hardware maintenance knowledge necessary
- Concerns over data security and network latency
When it comes to deep learning applications, specifically PyTorch, GPU-Mart is second to none. Specially designed to accelerate high-performance computing projects, GPU-Mart offers an impressive range of over 19 latest GPU models, unparalleled power, and speed.
GPU-Mart poses as an ideal hosting solution for projects involving artificial intelligence, scientific simulations, video rendering, and gaming. Users can expect reliable service and lightning-fast processing times, ensuring maximum productivity.
With a dedicated 24/7 technical support team, users are assured assistance for any challenges encountered. GPU-Mart also backs a 99.9% uptime guarantee, ensuring non-interrupted workflow.
The pricing is highly affordable, starting from as low as $21 per month. The cost-effective packages balance performance and affordability, presenting options like dedicated GPU servers with high parallelism, high throughput, and low latency.
As for customization, GPU-Mart allows users to select their preferred GPU, memory, storage, and more hardware configurations. This flexibility allows you to tailor the services to your computing power needs.
Overall, GPU-Mart brings to the table high-performing hosting solutions, affordable pricing, expert technical support, and an amazing range of GPUs, making it a preferred choice for users involved in CPU-intensive tasks.
Read more: GPU-Mart GPU Server Hosting 2024
2. Paperspace
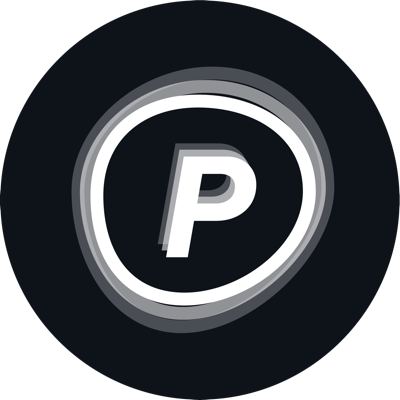
- Instance access to blazing fast GPU and IPs
- Low-cost GPUs with per-second billing
- Easy setup and always fast
- Paperspace provides a unified platform designed for your entire team
- Starting at $0.0045/hour
Pros
- Reliable performance and speed
- Value for money
- Flexibility and scalability
- Quick and easy setup processes
Cons
- Some issues with loading Conda environments
- Limitations of auto-shutdown feature
- Occasional customer support challenges
- Potential for billing concerns
Paperspace is well-recognized for offering state-of-the-art infrastructure for high-performance computing. Specifically, it has earned a reputation for providing access to some of the fastest and latest NVIDIA GPUs and IPs, making it an ideal choice for PyTorch users and AI developers.
The company’s adoption of low-cost GPUs with per-second billing allows users to save up to 70% on compute costs. The pricing is transparent and predictable – users only pay for what they use, with no commitments.
Paperspace offers a seamless onboarding process allowing users to switch from the setup stage to training models within seconds. The available pre-configured templates and the automatic versioning, tagging, and life-cycle management features ensure a fuss-free experience.
The platform’s scalability is noteworthy as it provides a range of GPU options with no run-time limits, making sure it effectively caters to varying user requirements. The collaborative features bring together resources and insights, improving team utility and overall efficiency.
Despite the benefits, some users have raised concerns over managing Conda environments, issues with the auto-shutdown feature, occasional customer service responsiveness, and potential billing issues, indicating areas that Paperspace could improve.
Regardless of these, Paperspace’s solid performance, user-friendly interface, impressive scalability, and cost-effective GPU-based PyTorch servers have contributed to it being a popular choice among AI developers and similar users.
3. OVHCloud

- Designed for processing massively parallel tasks
- Uses NVIDIA Tesla V100S GPUs optimized for AI and Deep Learning tasks
- Plenty of configurations with a range of memory and storage options
- Cloud-based solution perfect for scalable and remote workflows
- Starting at $0.77/hour
Pros
- User-friendly and responsive experience
- Scalability and customization
- Cost-effective, with no upfront costs for GPU servers
- Support for popular AI Frameworks like PyTorch
Cons
- Lack of specific CPU information
- Outdated hardware
- Customer service could be improved
- Possible learning curve
OVHCloud is well-known for the GPU servers it offers, specially crafted to handle massively parallel tasks. Its GPU servers are integrated with NVIDIA Tesla V100S CPUs, ideal for data-heavy workloads, including machine learning and deep learning tasks like working with PyTorch.
Benefitting from cloud technology, OVHCloud provides the flexibility of on-demand resource utilization while ensuring scalability, especially relevant for remote work scenarios and rapidly evolving workflows.
OVHCloud comes with a number of configurations in terms of memory, storage, and processor options, catering to a wide range of needs. The GPU servers offer a memory range from 45GB up to 180GB and storage capacities from 300GB to 2TB NVMe.
The GPU instances provided by OVHCloud directly transmit the power of NVIDIA cards via PCI Passthrough to the instance, without virtualisation, allowing users to harness the full potential of the hardware for their applications.
Moreover, OVHCloud has a strategic partnership with NVIDIA, resulting in a high-performing GPU-accelerated platform designed for deep learning and artificial intelligence applications, further supporting PyTorch workloads.
Despite the wide range of offerings and robust performance, the interface is designed to be user-friendly. Using OVHCloud services, developers can focus on their core tasks, leaving the infrastructure management to the professionals.
In terms of the pricing model, it starts at a very reasonable rate of $0.77/hour, making high-performance GPU servers accessible to even small start-ups or individual developers.
While OVHCloud comes with a lot of advantages, there are a few areas of concern as well. Customers have reported issues with customer service and initial setup, and the usage of outdated GPU hardware could be a potential limitation for some users. Nonetheless, OVHCloud’s offering is on par with several leading service providers in the market, offering capable GPU servers to facilitate PyTorch-based tasks and other similar workloads.
4. Cherry Servers
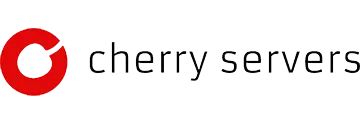
- Dedicated GPU Servers for high-performance computations
- Flexible server configurations with variety of GPUs
- In-memory computing with up to 1536GB RAM
- Secure data storage and private networking
- Specifically optimized for PyTorch
- Starting at $81/month
Pros
- Competitive pricing
- Reliable uptime and performance
- Responsive customer support
- Suitable for specific needs
- Supports Crypto Proof of Stake (PoS) Validator
Cons
- Limited SMTP services
- Delayed server availability
- Occasional issues with customer support
Cherry Servers is an excellent choice for dedicated GPU servers, especially for high-performance computations involving machine learning models and PyTorch applications. The deployment process for customized servers is quick and secure, with access available within 2 to 24 hours.
Raw compute power and the choice from 4 available GPU accelerators give users many options based on their specific needs. In-memory computing allows you to add up to 1536GB RAM to your server, ensuring your applications run smoothly.
Secure data storage options further enhance the safety of your data while the robust 10G virtual LAN assures smooth interconnection between servers. With up to 100TB of free monthly traffic, Cherry Servers offers impressive cost-effectiveness for users.
Cherry Servers offers a range of dedicated server plans with prices starting at just $81 per month, making it an affordable solution for businesses and personal use.
Feedback from Trustpilot reviewers highlight the good and affordable cloud servers, with minimal downtime and effective customer support. However, there are mentions of limits on SMTP services and occasional issues with customer support. Potential users should consider these factors when deciding on a hosting provider.
Despite a few minor drawbacks, Cherry Servers is highly recommended for its dedicated GPU Servers and hosting, especially for those who require high-performance computations and machine learning model training using PyTorch.
5. Google Cloud
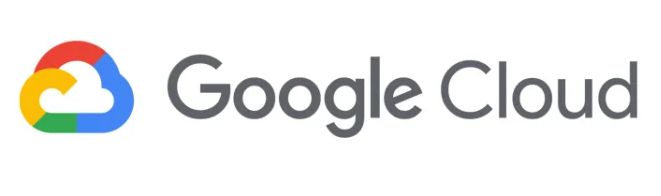
- High-performance GPUs for machine learning and scientific computing
- Wide selection of GPUs to match different performance and price points
- Flexible pricing and machine customizations to optimize workload
- Excellent storage, networking, and data analytics technologies
- Starting at $0.35/GPU
Pros
- Supports deep learning work
- Resource flexibility
- Availability of high-demand GPUs
- Ease of use
Cons
- Scarcity of GPUs, especially the high-demand A100 GPUs
- Availability issues in specific zones
- Quota limitations
- Lack of Transparency in Availability
- Limited access for individuals or small groups
Google Cloud offers high-performance GPUs that are perfect for machine learning, scientific computing, generative AI, and, in particular, running PyTorch scripts. They provide an array of GPUs with a comprehensive range of performance and price points, making it an ideal choice for projects of various scopes.
Google Cloud allows users to balance the processor, memory, high-performance disk, and up to 8 GPUs per instance for individual workloads. This level of customization delivers optimal results based on the per-second billing, ensuring users only pay for what they use.
Despite the high demand for A100 GPUs, Google Cloud tries to meet the needs of its users by providing these powerful GPUs whenever possible. Other GPU options like L4 are also available, ensuring users can carry on their work without disruption, even in the scarcity of high-demand GPUs.
Google Cloud offers excellent storage, networking, and data analytics technologies. This ensures that you have all the resources necessary to run a robust PyTorch server with ease.
There are some challenges to using Google Cloud’s GPU services. These include the scarcity of GPUs, availability issues in specific zones, quota limitations, and less transparency in availability. These issues often make it difficult for individual users or small groups to access the GPU resources, implying an advantage for larger organizations.
Notwithstanding these challenges, Google Cloud proves itself as a reliable choice for users seeking high-performance GPUs suitable for a wide array of tasks, including PyTorch-specific computational needs. With various GPUs available at competitive price points, Google Cloud offers flexible options to strike the right balance between cost and performance.