TensorFlow GPU hosting provides a powerful and efficient environment for training and deploying deep learning models. Leveraging the computational power of GPUs, developers and data scientists can significantly accelerate the processing of complex algorithms and large datasets.
This hosting solution is ideal for anyone looking to harness the full potential of TensorFlow for machine learning projects, offering scalable resources to meet the demands of both research and production environments.
Table of Contents
The Top 7 Best Tensorflow GPU Hosting
1. GPU-Mart
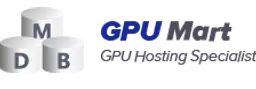
- Dedicated GPU hosting for high-performance projects
- Supports 19+ models of GPU cards, including latest Nvidia RTX series
- Supports major GPU computing applications like Tensorflow, Pytorch etc.
- 24/7/365 Technical Support offered
- Starting at $21.00/month
Pros
- Multiple GPU models available
- Supports a wide variety of computational tasks
- Customizable hardware configurations
- Flexible scalability options
Cons
- Needs careful budgeting due to specialty hardware
- Potential for high network latency
Gpu-Mart is renowned for its commitment to delivering professional GPU Hosting for your high-performance computing projects. It has expanded its support to 19+ models of GPU cards, offering unprecedented power and speed for even the most intricate applications. This makes it a perfect fit for Tensorflow users who can take advantage of GPU accelerated computing.
With robust infrastructure and the ability to optimize servers for lightning-fast processing times, Gpu-Mart ensures a remarkably quick processing experience for your projects.
The company utilises its state-of-the-art data centers in the USA to provide optimal speed experiences. Furthermore, their expert support team is available around the clock to resolve any technical challenges you might face.
When it comes to pricing and plans, Gpu-Mart offers a variety of options to cater to different project needs and budgets.
Their GPU hosting plans start at just $21.00 per month, providing an affordable entry point for individual developers and small businesses. Users can select hardware configurations to best match their needs and workload requirements.
For users looking for more advanced speed and performance, Gpu-Mart offers dedicated GPU server rental with various high-performance GPUs like Nvidia Tesla K80, RTX 3060, and more.
With its versatile use cases and customizable solutions, coupled with the promise of 99.9% uptime and affordable pricing, Gpu-Mart can be considered a great choice for professionals seeking a reliable and high-performing GPU hosting solution.
2. OVHCloud

- Optimized for massively parallel processing tasks
- Propelled by NVIDIA graphic processors
- ISO/IEC 27001, 27701 and health data hosting compliance
- Integrated with NVIDIA NGC for seamless container deployment
- Starting at $0.77/hour
Pros
- Highly performant GPUs for data processing
- Compliant with international security standards
- User-friendly interface with Docker config support
- Suitable for AI Training and Deep Learning
Cons
- Some users report challenging login process
- Hardware may be outdated in comparison to competitors
- Mixed customer reviews
- Data security and network stability concerns
OVHCloud is known for delivering cloud servers optimized for processing massively parallel tasks. Leveraging NVIDIA graphic processors, the hosting provider offers solutions that stand up to the demands of machine learning and deep learning applications.
With an impressive range of GPUs, including NVIDIA Tesla V100S, OVHCloud assures swift computation for intensive tasks. In addition, they also have a cloud-based architecture to assist users who want to avoid on-premise systems.
OVHCloud scores high on adaptability, offering varying configurations based on memory and storage requirements. Moreover, it has several layers of security compliance ensuring a secure hosting environment.
Their partnership with NVIDIA NGC makes deploying GPU-accelerated containers simpler, offering you the best-in-class GPU-enabled platform for artificial intelligence (AI), high-performance computing, and deep learning.
With straightforward pricing structure starting at $0.77 per hour,OVHCloud provides an affordable solution for developers and businesses requiring GPU resources for their projects.
Nonetheless, user experience with OVHCloud’s hosting may vary, and users might encounter challenges with the login process due to their unique website layout for different regions. Despite the potential drawbacks, OVHCloud remains a reliable choice for GPU-centric workflows and AI tasks.
3. Paperspace
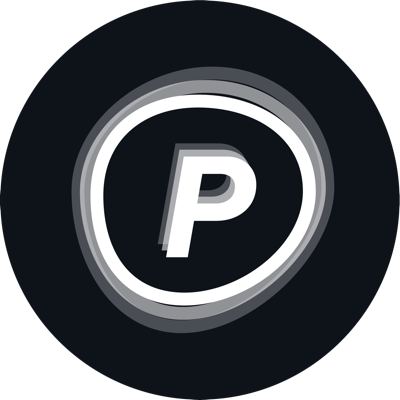
- Infinite compute power with access to top GPUs
- Lower costs with per second billing
- Hassle-free instance scaling and cancellations
- Pre-loaded with “ML in a Box” template for AI operations
- Starting at $0.0045/hour
Pros
- Ease of use with user-friendly interface
- Good performance and scalability
- Value for money
- Efficient customer support
- Fast setup and auto-shutdown feature
Cons
- Challenges with Conda environments in JupyterLab
- Limited auto-shutdown feature
- Some billing concerns reported
- Performance issues on lower-tier machines
Paperspace stands out in the hosting provider landscape for its immense computational power and affordable pricing. It employs state-of-the-art technology offering a vast catalogue of the latest NVIDIA GPUs, making it a fitting choice for AI developers and those handling computationally heavy tasks.
The provider guarantees seamless operations with its pre-configured templates and infrastructure abstraction, allowing users to kick-start their machine learning or GPU intensive tasks within seconds after signup. The compatibility with TensorFlow further enhances the platform’s capabilities and user experience.
Pricing with Paperspace is competitive and flexible, providing options from a mere $0.0045/hour. Moreover, with per-second billing, users can save up to 70% on compute costs compared to other public clouds or owning personal servers. The on-demand pricing structure means users only pay for the resources they use, ensuring cost efficiency.
Apart from computational services, Paperspace excels at offering user-friendly interfaces, efficient customer support, and a fast setup process. It also equips users with the auto-shutdown feature, automatically shutting down machines during inactivity to manage costs and energy effectively.
Despite some reported concerns related to the limitations of the auto-shutdown feature and minor billing issues, Paperspace largely earns user satisfaction for its robust performance, scalability, and value for money. It positions itself as a powerful instrument for AI developers and anyone requiring high-performance computing resources.
4. Lambda
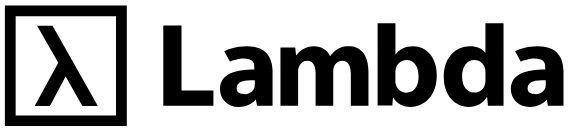
- Trusted by top companies and research labs
- One of the first providers to offer NVIDIA H100 on-demand
- Flexible multi-GPU instance options
- Lambda Stack used by more than 50k ML teams
- Starting at $2.49/hour for NVIDIA H100 instances
Pros
- Ready for use out of the box
- Cost-effective solution for machine learning applications
- Advanced technology for improved performance
- Diverse range of hosting options
Cons
- Pricier than some competitors
- Some reported hardware reliability issues
- No NVlink in dual RTX 3090 config
- Support quality can vary
Lambda has a sterling reputation for delivering top-notch hosting services, and is trusted by renowned global companies and esteemed research labs. Its technology powers various sectors ranging from business to scientific research.
Lambda equips you with some of the best hardware for machine learning tasks. Its Tensorbooks are a popular choice among data scientists for their ability to handle demanding workloads effectively.
On the cloud front, Lambda offers on-demand GPU instances, starting at $2.49 per hour. It takes pride in being one of the first cloud providers to make NVIDIA H100 Tensor Core GPUs available on-demand in a public cloud. Apart from that, they also offer a variety of options for reserved cloud instances equipped with NVIDIA H100s, H200s, and GH200s.
Lambda’s attractive suite of offerings also includes the Lambda Stack—a single-line installation and managed upgrade path for PyTorch®, TensorFlow, CUDA, cuDNN, and NVIDIA drivers. With this stack, Lambda has managed to support over 50,000 machine learning teams.
Their cloud resources certainly stand out as exceptionally customizable and have different types to suit varying workloads & budgets: 1x, 2x, 4x, & 8x GPU instances, optimizing flexibility and performance.
With a somewhat higher price point than some competitors and a few reported hardware reliability issues, Lambda is not without its cons. However, their commitment to providing top-tier hosting services, and a suite of products ideally suited for machine learning applications, makes Lambda a noteworthy contender in the tech arena.
5. Google Cloud
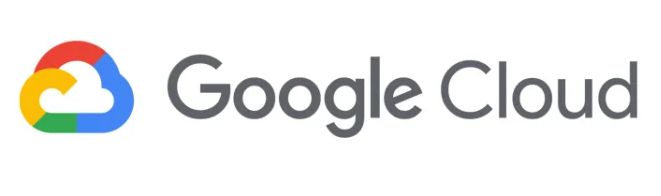
- Optimized for machine learning and scientific computing
- Wide selection of GPUs for different price-points and needs
- Flexible pricing and machine customizations
- Powerful and popular GPUs like A100 and L4
- Starting at $0.35/GPU
Pros
- Excellent for deep learning tasks
- Various types of GPUs available for different needs
- Great for both small and large projects
- Excellent accessibility and user experience
Cons
- High-demand GPUs may be scarce
- Restricted access in certain zones
- Possible quota limitations
- Lack of transparency in GPU availability
Google Cloud stands firmly as an exceptional provider of cloud services, with a focus on high-performance GPUs for tasks like machine learning, scientific computing, and generative AI.
With a wide selection of GPUs that encompass a range of performance and price points, Google Cloud offers adaptable solutions for users with diverse requirements. The platform is particularly renowned for having high-demand GPUs like A100 and L4 that are a boon for deep learning tasks.
The pricing model is flexible and caters to varying workload requirements, with users only paying for what they use. This is further complemented by machine customizations that allow for an optimal balance between the processor, memory, high-performance disk, and up to 8 GPUs per instance.
Despite the scarcity of high-demand GPUs, Google Cloud strives to make these powerful resources available, presenting customers with a plethora of alternative GPU options.
Focusing on ease of use, it’s straightforward to set up and use GPUs on Google Cloud, making it accessible for users with varying levels of expertise.
Another noteworthy feature is the complete support for TensorFlow, a powerful end-to-end open-source platform for machine learning. This makes Google Cloud a robust platform for running deep learning tasks.
While Google Cloud proves to be a formidable provider of cloud GPUs, it does face some challenges like the scarcity of GPUs, limited GPU availability in specific zones, potential quota limitations, and lack of transparency in GPU availability. Nevertheless, its versatile offerings and competitive pricing models demonstrate that it is a reliable choice for both individual developers and larger organizations.
6. TensorDock
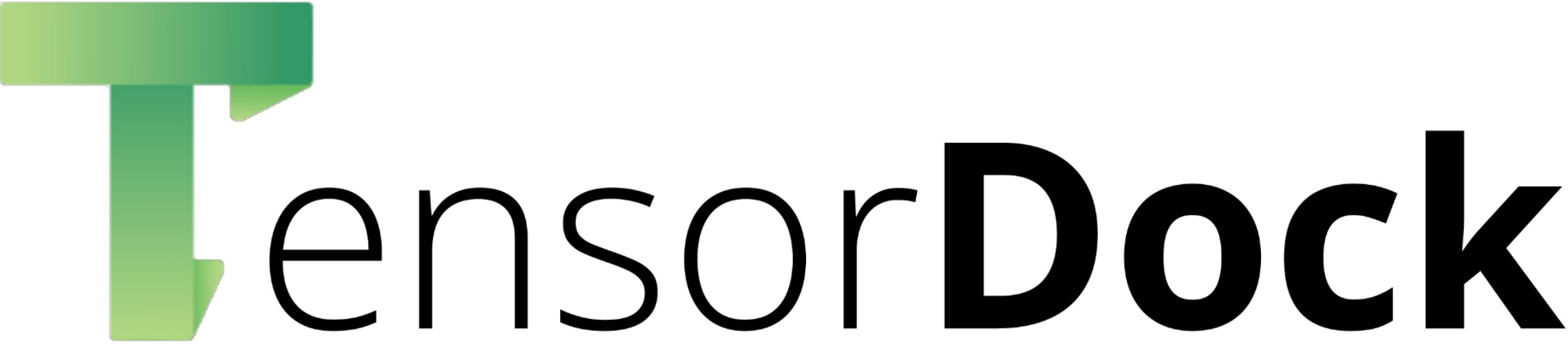
- 80% cheaper GPUs as compared to other cloud services
- Range of GPUs including NVIDIA T4s to H100s
- Secure and reliable with partners having proven uptime and security measures
- Fast VM deployment with multithreaded & optimized end-to-end service
- Starting at $0.05/hour
Pros
- Affordable rates and flexible configurations
- Pre-installed essential software eases the setup process
- Rapid provisioning and good performance for smooth gaming experiences
- Responsive support team
Cons
- Storage costs, even when VM is turned off
- Limited global reach with potential latency issues for non-US users
- May be complex for non-technical users
- Prepaid model and potential for additional costs
TensorDock is a customizable and flexible hosting provider that specializes in GPU-based services. Leveraging their competitive price structure, customers can appreciate 80% cheaper GPUs, which is an incredible deal compared to similar hosting providers.
Offering a wide range of GPUs from NVIDIA T4s to H100s, TensorDock ensures servers meet various needs, from gaming to image processing and rendering. Deployment is remarkably effective, with VMs provisioned in approximately 2 minutes and multithreaded optimizations to provide uncompromising speed.
The provider guarantees security and reliability as they partner with insulated suppliers having proven uptime and security measures. Their hosting is infinitely scalable with over 65,000 GPUs available through them and their hosts, perfect for growing businesses.
TensorDock has a strong focus on usability and convenience, with pre-installed Chrome and Parsec software and API access. They also go beyond usual hosting services by offering unique features like real-time stock checks, making the overall experience more controllable and user-friendly.
Although the service is primarily US-focused, resulting in latency issues for non-US users, it maintains a strong reputation for outstanding performance and smooth gaming experiences. It’s evident that for developers seeking a robust and affordable GPU-based hosted service, TensorDock should be a serious consideration.
7. CoreWeave
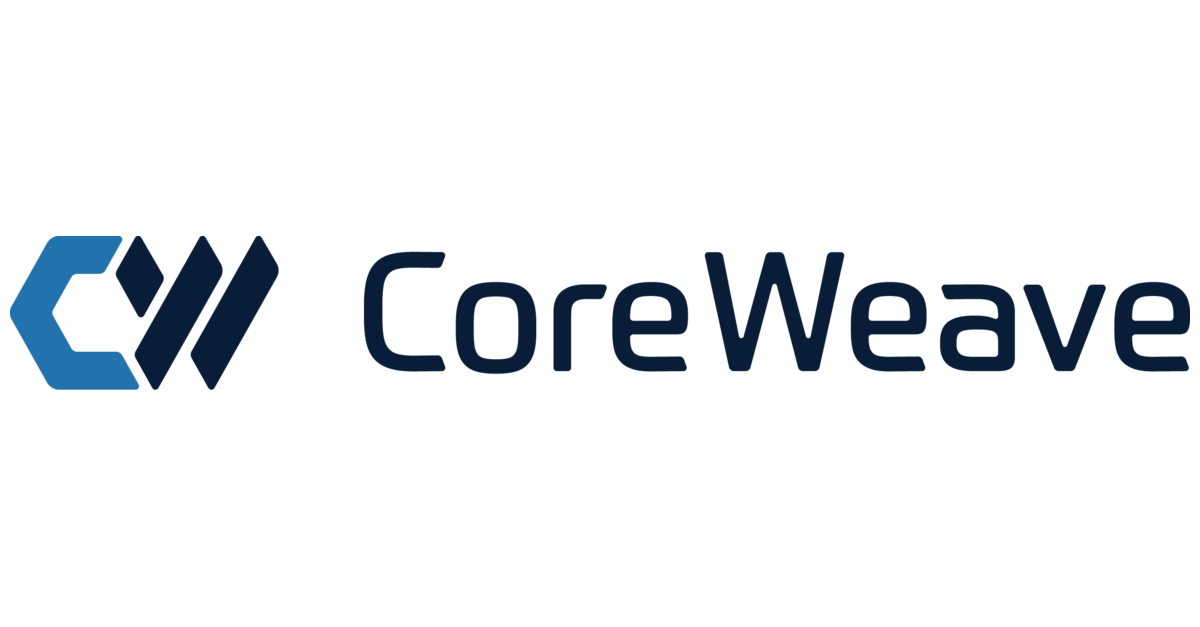
- Modern, Kubernetes-native architecture
- Significantly faster and cost-effective compared to traditional cloud providers
- Offering both GPU and CPU compute instances
- Impressive networking capabilities with no charge for egress
- Starting at $0.24/hour
Pros
- Purpose-built for large scale, GPU-accelerated workloads.
- Comprehensive storage and networking features
- Up to 35x faster and 80% less expensive than legacy cloud providers
- 14 Tier 4 data centers across North America
Cons
- May be additional costs related to storage and networking
- No significant discounts for long-term reserved instances
- Potential limitations in shared persistent storage
Specializing in GPU cloud hosting, CoreWeave positions itself as a key player in the industry by providing a massive scale of GPUs sitting on top of a robust, Kubernetes-native infrastructure. It’s suitable for a myriad of applications such as Machine Learning & AI, VFX & Rendering, and Pixel Streaming.
Experience unmatched speed for your tensorflow GPU hosting needs as CoreWeave claims to be up to 35x faster, enhancing your high-performance computing tasks with a 50% reduction in latency.
On top of speed, the cost-effectiveness of CoreWeave’s services makes it an attractive option. They are 80% less expensive compared to traditional cloud providers, ensuring you receive the most value from your investment.
With 14 Tier 4 data centers spread across North America, CoreWeave boasts an extensive network that guarantees robustness and reliability. Their expansive selection of different NVIDIA GPU SKUs can cater to a broad range of processing needs.
Moreover, CoreWeave also offers high-scale CPU-only instances for projects that don’t require GPU acceleration, featuring powerful Intel Xeon and AMD Epyc processors. With support for fully managed Kubernetes services, you can efficiently deploy containerized applications with the performance of bare-metal infrastructure.
The platform is not without its challenges. While the pricing for GPU services is competitive, there can be hidden costs related to storage and networking. Additionally, the lack of significant discounts for long-term use and potential limitations in shared persistent storage may be a drawback for some.
Despite these, CoreWeave continues to impress with its advanced storage solutions, comprehensive networking capabilities, and a modern infrastructure that supports a multitude of complex models and workflows.